In viticulture, grapevines face many threats, with virus diseases standing out as insidious adversaries. Among these, leafroll and red blotch pose significant challenges due to their elusive nature and lack of curative solutions. Traditional containment methods involve the laborious and costly process of identifying infected vines and replacing them with healthy ones (i.e., roguing). Identifying virus-infected grapevines is no simple task. It requires the expertise of individuals well-versed in the nuances of virus symptoms. Even for professionals, accurate diagnosis often necessitates sampling vine tissue for laboratory analysis, typically through molecular testing such as Polymerase Chain Reaction (PCR). This process is time-consuming and financially burdensome, underscoring the urgent need for innovative solutions to streamline detection efforts. Recent advancements in technology offer a glimmer of hope, promising more efficient and precise methods of detection and management.
Vineyard management has seen a surge in precision tools to optimize cultivation practices. Central to this trend is the development of sensors capable of delving deeper into the vine’s spectral response to stress. These advanced technologies empower growers to monitor vine health with unprecedented precision, offering insights that were once inaccessible through conventional means. In 2019, a groundbreaking project was launched at California State University, Fresno, funded by USDA, CDFA, CSU-ARI and F3, Fresno-Merced Future of Food innovative initiative, and in collaboration with experts from Cornell University and UC ANR. The initiative aimed to revolutionize the detection of leafroll and red blotch viruses using hyperspectral imagery.
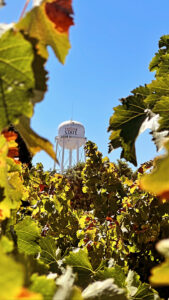
Hyperspectral Imagery
At its core, hyperspectral imaging is a cutting-edge technology that enables measuring an object’s response to light absorption across a wide range of wavelengths. By analyzing the spectral signature of grapevines, researchers can glean valuable insights into their physiological and health state. For instance, variations in chlorophyll and anthocyanin concentrations manifest as distinct patterns in the visible spectrum (the portion of light wavelengths we interact with our eyes), while changes in cell structure influence near-infrared wavelengths (which are not visible to our eyes). Furthermore, water content and other chemical compounds (cellulose, sugar) leave their imprint in the shortwave infrared domain. Crucially, diseases such as leafroll and red blotch can alter these spectral signatures, offering non-invasive means of detection.
The project’s first phase involved sampling 500 leaves from both healthy and virus-infected plants (by leafroll, red blotch or both viruses). These leaves were then subjected to hyperspectral imaging within the controlled environment of a dark cabinet in the laboratory, capturing images across the 500- to 700-nm range. Machine-vision techniques, particularly Convolutional Neural Networks (CNN), were employed to analyze hyperspectral imagery and detect signs of infection in grapevines. CNNs are a type of deep learning algorithm that excels at image recognition tasks by automatically learning features from raw pixel data. During this phase, the CNN model achieved impressive results. For binary classification, distinguishing between infected vines (with red blotch or leafroll) and healthy ones, the model attained an accuracy of 87% when symptoms were visible at post-veraison and 85.6% when symptoms were not yet visible to the naked eye, thus at pre-veraison. This demonstrated the model’s effectiveness in detecting infections even before visible symptoms manifested. Furthermore, in a more complex multiclass classification task aimed at identifying the specific virus infecting the vine, thus distinguishing between leafroll and red blotch, the CNN model achieved an overall accuracy of 76.9%. This highlights the model’s ability to differentiate between viruses based on subtle spectral variations (Sawyer et al. 2023).
Buoyed by these promising outcomes, the project advanced to its second phase, transitioning from the controlled environment of the lab to the dynamic conditions of the field. Utilizing the same hyperspectral camera, images of the side of 700 vines were captured in the vineyard, focusing specifically on detecting red blotch virus. When symptoms were not visibly apparent, a model achieved an overall accuracy of 68.6%. As the season progressed and symptoms became more pronounced, the accuracy improved significantly, reaching 76.6% with a Support-Vector Machine (SVM) model. This underscores the model’s adaptability to real-world conditions and its efficacy in detecting infections even in the absence of visible symptoms (Laroche-Pinel et al. 2024a).
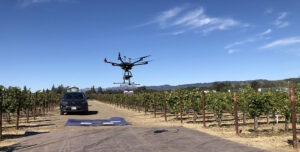
In the third phase of the project, the approach was elevated by deploying a drone-mounted hyperspectral camera to capture images of ~300 vines during the post-veraison stage. This aerial perspective provided a comprehensive view of the vineyard, enabling it to assess vine health and identify infections across a potentially larger scale. Expanding beyond mere detection, the focus shifted toward leveraging the spectral response of the vines to extract valuable biochemical information. Specifically, a model was used to predict the concentrations of three key pigments (chlorophyll, carotenoids and anthocyanins) based on the spectral data captured by the drone. The striking results revealed significant differences in pigment concentrations between infected and non-infected vines. Non-infected vines exhibited higher predicted levels of chlorophyll and carotenoids, indicative of healthier foliage and photosynthetic activity. Conversely, infected vines displayed elevated levels of anthocyanins, a response often triggered by stressors such as viral infections. Utilizing the spectral information, machine learning techniques were applied to classify vines as either infected or non-infected by red blotch. The model achieved an impressive accuracy rate of 87% in binary classification, further underscoring the efficacy of hyperspectral imaging coupled with advanced data analysis in disease detection and management (Laroche-Pinel et al. 2024b).
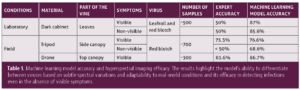
Pivotal Advancement in Vineyard Monitoring
This phase represents a pivotal advancement in vineyard monitoring, offering a holistic approach that transcends the mere identification of infections. By harnessing the spectral signatures of grapevines, growers gain valuable insights into their physiological status and biochemical composition, empowering them to make informed decisions regarding vineyard management practices. The project’s third phase marks a significant milestone in the quest for more effective vineyard management strategies. Overall, these findings represent a significant leap forward in vineyard management. As the battle against grapevine virus diseases rages on, the integration of hyperspectral imaging holds immense promise for vineyard management. By harnessing the power of advanced technology, growers can detect and mitigate threats more effectively, safeguarding the health and productivity of their vineyards. With ongoing research and innovation, the vision of a future where precision tools enable proactive disease management is within reach.
References
Laroche-Pinel, E., Singh, K., Flasco, M., Cooper, M.L., Fuchs, M., Brillante, L. (2024a). Grapevine Red Blotch Virus Detection in the Vineyard: Leveraging Machine Learning with VIS/NIR Hyperspectral Images. (In review)
Laroche-Pinel, E., Singh, K., M., Cooper, M.L., Fuchs, M., Brillante, L. (2024b). Advanced Detection of Grapevine Red Blotch Virus at the Plant Level in Vineyards: A Drone-Based Approach using VIS/NIR Hyperspectral Camera, Machine Learning, and PROSPECT Inversion Model. (In Prep)
Sawyer E., Laroche-Pinel E., Flasco M., Cooper M.L., Corrales B., Fuchs M., Brillante, L. (2023) Phenotyping grapevine red blotch virus and grapevine leafroll associated viruses before and after symptom expression through machine-learning analysis of hyperspectral images. Frontiers in Plant Science. 14:1117869. doi: 10.3389/fpls.2023.1117869